Seasonal forecasts
Seasonal forecasts
Weather changes on a daily basis. While modern forecasting systems predict accurately the weather a few days into the future, daily predictions beyond two weeks are mostly useless. However, it is possible to predict average climate trends covering up to one year in advance, through seasonal predictions. In contrast with the popular weather forecasts, that provide specific outputs (e.g. temperature will be 15.5 ℃ in Madrid tomorrow), seasonal predictions provide information on how seasonal average weather is likely to be a few months in advance (e.g. there is a 80% chance that the next season will be hotter than usual in central Spain).
Agriculture, energy, transport... Businesses from many different sectors are already incorporating science-based climate information into its planning, production, safety and logistics decisions, especially to plan ahead of the coming season. Despite their potential value, seasonal forecasts are currently underexploited due to some limiting factors. In order to cope with these limitations, we provide capacity building and tailored evaluation of seasonal predictions for your business, saving time and money.
Need more information? Read our brochure on seasonal forecasting, or read below for specific details on what we offer.
Key features
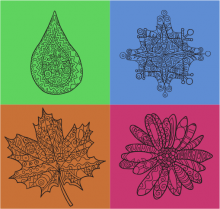
What we offer
Due to the seasonal predictions' complexity, we offer different services:
- Capacity building and consultancy: we offer training and consultancy services that go from one day to one week, adapted to your needs. These will describe the scientific basis and the state-of-the-art of seasonal forecasting, and allow you to interpret the existing products and their associated uncertainty.
- Tailored evaluation of seasonal forecast skill: we provide proper evaluation of forecast skill (accuracy levels) for targeted areas and variables. This process takes into account the retrospective predictions of the top global climate models for the last two or three decades, as well as appropriate statistical validation scores that account for different aspects of a forecast.
- Operational tailored predictions: we provide operational predictions for your business, based on the best performing global models and calibration tools. A pilot application, applied to fire hazard management, can be consulted on this seasonal forecasting of Fire Weather Index (FWI).
- Local calibration and adaptation: we are specialists in applying statistical techniques which properly adapt the coarse-resolution global seasonal forecasts to your locations of interest.
Validation of seasonal forecasts
Since seasonal predictability varies strongly across regions and seasons (see [1] [2] [3] [4] for more information), validating the forecast quality is and essential part of the proccess. This validations quantifies the uncertainty associated to a forecast for a particular location and time of the year.
These validations are based on the inter-comparison of the forecast outputs with the past climate, through different methods. To provide a standardised validation process, we follow the Standardized Verification System for Long Range Forecasts (SVS-LRF). This framework defines a suite of standard metrics that allow forecasting centres to document the quality of their forecasts.
Based on this standard, and depending on the final use of the forecasting and its sector, we offer different metrics for verification:
- Deterministic: Mean Error (or bias), Anomaly Correlation Coefficient (ACC) or Root Mean Square Error (RMSE).
- Probabilistic: Ranked Probability Skill Score (RPSS), ROC skill score (ROCSS) or Reliability diagram
Product limitations
Despite its potential value for many sectors, seasonal forecasting remains currently underexploited due to several limitations:
- Seasonal forecasts are probabilistic: hence, there is a difficulty to correctly interpret this kind of information and to properly incorporate probabilistic forecast products into business decision-making processess.
- The performance varies accross locations: the accuracy of the prediction is higher between the tropics, but the skill levels (accuracy) vary greatly depending on the predicted variable, region and season of interest. As a result, a robust tailored assessment of these products (based on past performance) is needed to explore their potential for each particular application.
- Coarse spatial resolution: Current spatial resolution for seasonal forecasting is 50-100 Km, which is too low for some practical applications. Therefore, post-processing is needed, using proper statistical calibration or downscaling methods that enable to go from global to local, obtaining suitable predictions.
References
[1] Halpert MS, Ropelewski CF. 1992. Surface temperature patterns associated with the Southern Oscillation. J Clim 5:577–93. Link
[2] van Oldenborgh, G. J. 2004. Assessing the skill of seasonal forecasts, Tech. rep., KNMI Research Biennial Reports, 2003/04. KNMI. Link.
[3] Doblas-Reyes, F. J. and Weisheimer, A. and Palmer, T. N. and Murphy, J. M. and Smith, D. 2010. Forecast quality assessment of the ENSEMBLES seasonal-to-decadal Stream 2 hindcasts, Tech. Rep. 621. Link.
[4] Manzanas, R. and Frias, M. D. and Cofiño, A. S. and Gutierrez, J. M., 2014. Validation of 40 year multimodel seasonal precipitation forecasts: The role of ENSO on the global skill, Journal of Geophysical Research: Atmospheres, 119, 1708–1719, doi:10.1002/2013JD020680. Link.
[5] Jolliffe, I. T., and D. B. Stephenson, Eds., 2003. Forecast Verification: A Practitioner's Guide in Atmospheric Science. John Wiley and Sons, 254 pp.